From AI to deepfakes: How machine learning is affecting cybersecurity
Dr. Tsukerman graduated from Stanford University and UC Berkeley. He began his cybersecurity career in a small startup as a cybersecurity data scientist, where he developed a machine-learning-based anti-ransomware solution that won the Top 10 Ransomware Products award by PC Magazine. In addition, Dr. Tsukerman designed a machine-learning malware detection system for Palo Alto Network's firewall service, securing over 30,000 enterprise customers in real time. He is the author of the "Machine Learning for Cybersecurity Cookbook" and the popular Infosec learning paths "Cybersecurity Data Science" and "Machine Learning for Red Team Hackers.
– Get your FREE cybersecurity training resources: https://www.infosecinstitute.com/free
– View Cyber Work Podcast transcripts and additional episodes: https://www.infosecinstitute.com/podcast
Transcript
Chris Sienko: We recently hit yet another huge milestone here at the Cyber Work Podcast, 25,000 YouTube subscribers. Thanks to all of you who watch and listen each week, to those of you who watch the YouTube videos go live and chat with other and comments and everyone who is helping us to grow this great community.
To give back, we’re now giving you 30 days of team training for teams of 10 or more. Your Infosec Skills account will help your entire team develop their skills and earn CPEs through hundreds of IT and security courses, cloud hosted cyber ranges, hands-on projects, skills assessments and certification practice exams. Plus, you can easily monitor, assign and track training progress with team admin and reporting features.
If you have 10 or more people who need skills training, head over to infosecinstitute.com/cyberwork or click the link in the description to take advantage of the special offer for Cyber Work listeners. Thank you once again for listening to and watching our podcast. We appreciate each and every one of you coming back each week. On that note, I’ve got someone I’d like you to meet. So let’s begin the episode.
Welcome to this week’s episode of the Cyber Work with Infosec podcast. Each week, I sit down with a different industry thought leader and we discuss the latest cyber security trends, how those trends are affecting the work of infosec professionals while offering tips for those trying to break in or move up the ladder in the cyber security industry.
Our topic today is AI and machine learning and it’s going to be an exciting, if possibly a scary one. We’re heard all about deepfakes, and if you haven't, go look it up. Through a combination of incredible processing power and some rudimentary artificial intelligence technology, a lot of ingenuity and deviousness hackers and others, other bad actors are able to curate very realistic videos of human beings that appear to be saying unexpected, scandalous, seemingly impossible things.
Well, there are telltale signs that something's up, viewers looking to have their biases confirmed may not go through a mental checklist before sharing this type of harmful and blatantly false info through social media. This type of information can be especially catastrophic as we move into another election year.
Emmanuel Tsukerman is a professor of data science and machine learning. He’s created several courses for our infosec skills platform on this very topic. We’re going to talk today about the deep learning applications of cybercrime as well as some of the ways the same types of technologies can be used to counteract these threats. We’ll also be talking about the data science and machine learning courses that Emmanuel has created for infosec skills and how they can help your security career to rise to the next level.
Emmanuel, welcome to Cyber Work.
Emmanuel: Thank you, Chris. It's an honor to be here in Cyber Work.
Chris: Thank you so much for your time. I want to start out by asking you a bit about your security journey. How did you first get interested in computers and tech and cyber security and when did your attention turned to topics around AI and machine learning?
Emmanuel: Actually, I started my journey outside of cybersecurity focusing on statistics, machine learning and applied math more broadly. Then I got into cybersecurity because I knew it's a great place for disruption and it's always dynamically evolving. It's never going to be a boring field to work at.
Chris: And you found that to be true I assume.
Emmanuel: Yeah, especially –
Chris: Yeah. Yeah, could you –
Emmanuel: Developments of –
Chris: Okay. Could you give me sort of a compressed version of the projects, positions and training that took you from your early career to your current work as an educator and security consultant? What were some major educational experientials or project-based touchstones that, shall we say, made you what you are today?
Emmanuel: Yeah. When I first got out of school, I joined a small startup of four people, and the startup was focused on IoT security. At that time, IoT was exploding. It was kind of early, and we went on customer interviews asking large IT hospital, IT managers what are their primary concerns? What kind of products would be best for them? What's their interest? We learned that they’re actually concerned about – All of them were concerned about ransomware.
Then we decided why don’t we incorporate some ransomware aspect in our IoT suite? I worked on this project. I was sort of the new person, so I was assigned to this project, and the first question that I had was, “Why is ransomware a problem because there is antivirus, right? And ransomware is a virus.”
What I learned at that time, this was a few years ago, AVs mostly relied on signatures and heuristics. Signatures like kind of like a catalog to be very simple about it, and heuristics like rules that people come up with that are likely to work. But the problem was that every day you get like hundreds of thousands of new samples and there's no way these techniques can keep up, and the bad guys that are constantly innovating with new types of ransomware, and new techniques, new ways to penetrate, it’s always a problem. We decided since these approaches don't work, heuristics and signatures, maybe this is a perfect application for machine learning.
Having a data science background, I decided, “Let me try it out. Maybe it’ll work.” I mean, it sounds promising. Machine learning should be able to learn from the data. It should be able to learn from history and then figure out new samples that we haven't seen before, which is the issue we are facing.
I developed a virtual lab. I collected samples, conducted experiments, and eventually we did prototype a machine learning product, like a machine learning prototype, and we tried it out, we demoed it and decided we’re going to pivot completely from IoT to ransomware. Then we went ahead and developed this product and it was quiet successful. We had customers in large enterprises. We got awards and so on, and that was my first experience in cybersecurity, was like this – I didn't know what to expect. I come in for IoT. I shift into this experimental thing of ransomware, and it’s very exciting. I also worked at Palo ... Yeah, go ahead.
Chris: Oh! I was going to say, it sounds like you came into the sort of birth of ransomware fairly early too. That was pretty much in a newly developed thing at the time. Was that sort of part of the sort of opportunity was that no one else was really sort of working in this area?
Emmanuel: Yeah, it was right before WannaCry. It was like after some ransomware was out there, people are afraid, but it wasn't – It was before like the really big things happened, like WannaCry and NotPetya.
Chris: Got you. I want to talk today a little bit about deepfake, because on the infosec blog, you wrote about how deep learning and deepfakes are changing the face of cybersecurity. This is of course a huge subject right now especially this year, and you note that because of the rise of this technology, you said, “The ability of basic human intuition to prevent attacks is at an all-time low, whereas the need for training and specialists in cybersecurity is at an all-time high.” That’s obviously a scary stuff, but you also note that machine learning can be used as a countermeasure, and to quote you, “For every tool that utilizes deep learning for offensive purposes, there is a tool that uses deep learning to benefit humanity.” Can you tell us something about some of these counteroffensive tools that use this emerging technology to bring the fight to the bad actors?
Emmanuel: Yeah, for sure. Let me start by explaining why I think human intuition is now at an all-time low. There’s the recent crime where fraudsters, they manage to get a company. There is like a child company and a parent company and they got the child company to transfer $250,000. The way they did it was they pretended to be the CEO of this larger company using voice transfer artificial intelligence technology. It's like deepfake, but for voice. Like maybe I take your voice, Chris, and I say something using your voice and now I convince people who know you that you are saying it and therefore it must be this way. They indeed managed to do this.
I think the most disturbing part is that – I mean, this person, the victim was the CEO of the child company. He knew the CEO of the parent company. It's not like complete strangers and you’d think that maybe intuition would work here, but no. I think that if he was more aware of this possibility, I mean, maybe he had some nagging doubts like, “Does that make sense for the CEO to ask to transfer 250,000 to this unknown place? Well, no, but it is his voice, right? I guess it's him.” But if she knew that now we have technology that allows you to imitate someone's voice, then presumably he’d be much more cautious. You double check if that really makes sense. Maybe call up the CEO of the parent company and see that it's not him.
Now there are a lot of tools out there almost for every – I guess I’d call it like a forgery technology. There is like a counter technology that can detect when it’s forgery. There is for instance technology for detecting deepfakes. I guess we haven't defined deepfakes yet, but basically the most common instance is when you take a video of someone, let’s say a video of me talking and you replace my face with someone else. It can be a political figure. It can be maybe you replace a criminal’s face with like someone else and then you’re now basically getting someone else to take the fault. It's shockingly convincing. I mean, I've seen videos of this and I can’t tell. Is it deepfake or is it the real person? I don’t know. This is the deepfake technology. But yeah, there is technology for countering it, like you feed it into a neuronal network and it can tell you is this fake or isn’t real. The same applies for a lot of other things that are becoming more forgeable, like phishing, voice and so on and so forth. There are these technologies out there. Another approach is also reputation scoring. There is a lot of rising reputation score, like risk scoring using AI that can tell you if it's likely to be a scam or something like that.
Chris: Okay. Now, can we talk about some ways that deep learning could affect cyberattacks whether to enhance social engineering or stock market manipulations for example? I was going to say, since we don't know what we don't know, could we talk a little bit about some of the different possible types of deep fake enhanced attacks that we should be watching out for? What are some of the areas that deep fakes are most likely to be used in? Like I said, I mentioned that you could get a voice saying sell all the stocks or you could get a social engineering thing saying, “Hey, it's Chris. I'm in trouble. Send me money, dad,” or whatever. What are the sort of like main areas where deep fakes could really sort of do a lot of damage that you see?
Emmanuel: Yeah. You mentioned – I think one of the most important ones is phishing. This is for the common person everyday life. You can be concerned about voice impersonation. Maybe if someone is conferencing you, you don't really know what is happening behind the screen if it's – There has been demos where people do deepfakes. For instance, for all we know, this isn’t my face. Maybe I’m like anonymous, right? Put on different faces or something like that.
There is another area of huge concern for I guess everyone, which is the elections. You can imagine a broadcast of one of the candidates or someone related saying something and people wouldn't know that it's a fake.
In the blog post, I show a short video where they have on the former President Barack Obama saying some nonsense, but until he says the nonsense, like it starts out very formal and you think that looks real. Then you see a different person was like a puppet master who’s talking in his stand. Those are a few of the critical ones.
Chris: Right. Do you have any sort of tips for people sort of watching out for this kind of thing? Like I said, I know most people are going to want to use their confirmation bias and say, “Well, this person says it and I didn’t like it. I don't like this person.” So therefore they probably said it. But I've seen for example people say that like you can check like the hairline sometimes is a little off or the way the eyes move or stuff like that. Do you have any kind of sort of aesthetic tips in terms of watching out for this kind of thing?
Emmanuel: Yeah. Two thoughts on this. One is, yeah, it’s usually at least in the technology that’s been commonly used in the past few years. You could see like the face, like place here, and there might be a tiny bit of problems in the connection here. That's where you would see that it's a different thing. But nowadays, it’s becoming much more convincing and there are even – I forgot to mention that you can have deepfakes that aren't just the face, so that you can deepfakes through the whole body. Kind of deepfakes for like colors, like car color, color of the shirt I'm wearing. You can replace license plates, titles and so on and so forth. I think that's also an area to keep an eye out for. It’s not just the technology itself becoming better, like easier to generate a deepfake and the little artifacts of deepfake and being harder to see, but also the applications. People are coming up with very clever ones where you wouldn't know that they're doing it.
Chris: Yeah. What are your thoughts on how to combat this? Because I mean it sounds very apocalyptic in the sense that I can't really think of any way in which we’re going to sort of be able to strut this down. What are your sort of tips or advice coming into this election year whether legislative or just individual person? What do we do?
Emmanuel: Yeah, it’s a good question. When I think about this, it feels kind of like I'm riding almost like a science fiction novel. It's like – In the future, all videos will be the deeprakes. You won’t be able to tell if it's a real or not. Audios will be. It’s kind of like this sort of like Blade Runner thing. But I think that – This is just my thoughts, is that in the future, we’re going to cryptographically sign videos. There will be like a certifying authority, just like you have free internet or something like that and who would basically trust someone. Maybe if it's the US government that releases a candidate’s introduction or whatever it is, and there is like a cryptographic signature that you can verify for yourself and say, “Okay, that is the US government. No one is fooling me.” Whereas if it's a different country that's releasing the video for that candidate, there won't be able to verify. That's my thinking about that. I think there’ll be like to types of media, like the certified with crypto, cryptographic signatures, and then stuff that you can't verify that no one knows if it's true or not. Maybe it's more for entertainment.
Chris: Right? Do you foresee –
Emmanuel: I think we will get over it. There’ll be – Yeah, I was going to say. I think there’ll be a time where there’ll be confusion, some fear and a need for people to step in and then come up with solutions, which I suggest that, one, we’ll see if it's viable or not.
Chris: Right. That was going to be my question, was whether or not such a device is currently on the market or whether it's something that we’re still sort of speculating about at this point.
Emmanuel: Yeah. I think the technology is there. It's more of the desire to do it.
Chris: Right, the rollout.
Emmanuel: But its remains to be seen.
Chris: Yeah. You also mentioned an interesting blog post on our site about using machine learning applications to intercept and negate ransomware before the worst happens, and you mentioned a little bit of that at the start of the show. Could we talk more about that technology? What exactly are these sort of machine learning enhanced tools doing to sort of stave off ransomware before the worst happens?
Emmanuel: Yeah. Sure. Let me start with maybe how you don't detect ransomware. A naïve approach is to come up with some rules, like you study ransomware and you see, “Looks like ransomware likes to change extensions.” Maybe I'm going to come up with a rule where if a process changes the extensions of five files, say, and these extensions are not known, then let us kill this process, quarantine the file that produced it and declare it to be ransomware, and this is like your rules, like rule A.
But then the problem is what if this ransomware for instance takes the files, copies them, encrypts a new thing and it encrypts a copy and then erases the original. Then it got through your heuristic, or what if it encrypts a bunch of them at once? Again, you have a problem. You have to keep it in account that you don’t produce false positives, like legitimate applications that you've flagged.
Then you think of ways that are better, and the solution is machine learning, which I would say the definition is like automatic production of rules using large data and at scale. We came up with like one rule that didn't quite work, but we can let the data come up with rules itself and at scale and then we have a solution for ransomware. In that sense, you basically let the data come up with the rules instead of trying to keep up and having many people try various rules and then they get out of date a month later because hackers are privy to what people are up to. Then you have a solution that's also able to catch future attacks, like zero-day attacks, because the –
Chris: Okay. Moving on to the heart of the talk today, I want to talk to you about your work with infosec skills, our skills-based platform. Could you tell us about your cybersecurity data science skills courses and how they relate to this very current security concern?
Emmanuel: Yeah. I am often keeping an eye out on the market and what's going on, and I see a lot of disruption and innovation coming from machine learning in particular in cybersecurity. For instance, you’ll see like a startup that claims to have, “We have a solution to phishing,” and it relies on machine learning to maybe create a reputation score or maybe it’s an amalgamation of different solutions altogether.
I think for – I guess for job applicants and other people in cybersecurity, when you are applying and if you have a machine learning or data science experience, then you are an asset, because the hiring manager looks at it and maybe imagine we have like two candidates, one with the machine learning skills and one without and they see like the person with machine learning skills could be potentially helpful in designing this new solution that we are working on. This doesn't only apply to startups. There is also a lot of disruption in larger enterprises because they are aware that there are all these startups coming up that are disrupting, so they need to disrupt themselves and they are also hiring a lot of data scientists and machine learning practitioners in order to develop these new products. That’s also kind of my reasoning from a market perspective why I think it is very useful to have these skills aside from the practical usage of creating better solutions to cybersecurity problems.
Now, going in more details to the learning paths, the goal is to basically give people the fundamentals, the background, so that they can afterwards specialize in what they need, because you never know, like maybe you need to specialize in phishing and that’s something you’ll have to research. But if you have the basic tools in machine learning, you will apply your domain knowledge from phishing and you apply your tools to the domain of fishing.
Chris: Right.
Emmanuel: I cover a lot of things, but in particular I cover how much machine learning is used for malware detection. I go in a lot of depth here on feature engineering, the collection of the data. I talk about end-to-end machine learning, which is basically where you just let the neural network figure everything out by itself. That’s kind of like an amazing new thing.
I talk about how to use machine learning for intrusion detection. That is things like IDSs that rely on data science and data, and I talk about social engineering. So that’s like deepfakes, performing OSINT automatically. For instance, like having your machine learning system extract the text from videos and then searching through the text to find what you're looking for. For instance, maybe you want to know someone's, let's say, Twitter, Twitter account, and then you perform and utilizes this text extraction to find the keyword Twitter and then that helps you automate the search through all the person’s videos, which could be thousands. Okay. That's the cybersecurity data science learning path.
There is some more advanced learning path, which is machine learning for red team hackers. Here I go in depth on pentesting, and I do it from both sides of the equation. How to use machine learning to attack and how to use machine learning to prevent attacks? Also how to attack machine learning systems? I show how to do deepfakes from beginning, from A-Z. I show how to attack machine learning systems for the purpose of red teaming. I show how to do smart fuzzing.
I think you had a guest not too long ago discussing fuzzing. I show how to use machine learning to take it to the next level so that your fuzzer is learning from what it’s seeing. These are really powerful techniques, and I think I cover such a broad range of topics that anyone who’s done these learning paths has like a very good background. They can probably find similarity between whatever problem they're working on and something that you learn in the course.
Chris: Great. I want to talk a little bit about something. Whenever we talk about AI and machine learning on the show, and we’ve had couple other guests that have talked in similar areas, we get comments and questions about the possibility that improved automation process will replace low-level cybersecurity careers. Is that a real fear or should professionals in the cybersecurity ladder be considering these factors when choosing their career in the long-term or is it more sort of an add-on to sort of human element?
Emmanuel: I think this has been a fear that's kind of always been with us in the sense that we've always been concerned of getting automated out of our jobs. I think that applies to everyone, but I don't see it as a problem. I think machine learning is going to be more of a tool that enhances your ability, kind of like a computer, like you can do whatever a computer does. If you are filling out data, you can do it on pen and paper, but in the computer, it’s faster.
I think the same would apply for, let's say, security analysts. If they come up with rules of they’re looking at the SIM or something like that, well they can like 10X or 100X what they're doing by letting a machine learning system come up with more rules, better rules, or check the rules that they have. Similarly, if you are looking at SIM or SOC, you might use a machine learning system to focus your attention so you don't have to look at so many events, but maybe the system will tell you, “These are like the top hundred events. Focus your attention here.” Because the machine learning system is obviously not as intelligent as humans are. We are aware of many more things, the context, the background and so on and so forth. It makes sense to use it to kind of whittle down the easy stuff and bringing to our attention the important stuff that requires humans to solve.
Chris: Yeah, that’s really worth noting in the sense that a lot of people think that it's going to take away sort of humans, but it can't only take away human intuition. All it can do is add more computing power. A lot of the sort of problem solving is sort of having a human interpreting what another human is doing on the other side, and that's always going to be necessary I suppose.
Emmanuel: Yeah, definitely.
Chris: What can students taking these machine learning and data science courses expect to learn? You talked about it a little bit, but what types of career or professional specializations will be best utilized by learning about machine learning as you’ll be teaching it? Where would you start once you sort of have this information under your belt?
Emmanuel: I thought about this question, and I think that it is – These skills are especially useful for practitioners, people who are developing things, like for instance security analysts who need to come up with better rules, or maybe people who are looking to catch malware to improve the malware detection capabilities in their organization. But I think another section of people that benefits a lot from this understanding is actually the decision-makers, because they’re the ones who will decide what should we invest in? Should we purchase this tool? Should we do this training? Should we develop this new machine learning tool? How difficult would it be? It is practical? Can it perform better than what we have? These types of questions I think can only be answered by getting this type of knowledge by going through the course and seeing what does it take to create a machine learning classifier? Is it 10 years or is something can be done in one year? How much data? So on and so forth. I think decision-makers are really important people to learn this.
Another section of people would benefit is I think kind of the general public in the sense of being aware of the dangers out there, like we mentioned deepfake and voice transfer. Maybe I imagine they wouldn't need to go into as much detail. They don’t need to go through creating a malware classifier, but it sure doesn't hurt for them to see how deepfakes are made. How easy it is? I can make a deepfake in one day. Take your video and, “Bam! Deepfake.”It will be very useful for anyone in general to be aware of how machine learning is being used in cybersecurity.
Chris: In your opinion, what are the cybersecurity skills that are currently most in demand and most likely to accelerate your career? Are there skills, either the ones in your class or that you see out in the world that people are overlooking in their studies and preparation?
Emmanuel: From my perspective, I’m looking – I look a lot at like the disruptive elements, and for me it’s things like machine learning and various applications of it. I also keep – I look at the cybersecurity news very frequently on a daily basis and I keep seeing patterns, like ransomware is still an issue and it's actually attacking hospitals at this dark time, which is pretty sad. But that’s suggesting me that there is – I mean, obviously, there is – Since an attack has occurred at a hospital, there is a need for someone to have prepared better to prevent it or to at least be able to remediate it better.
Maybe auditing, better auditing seems to be required. Maybe just more people in cybersecurity.
Chris: I want to talk a little bit sort of platform of what we’re doing. As online training becomes more ubiquitous, what are your thoughts on skills-focused training versus boot camp or four-year academic subjects for your institution? What are the benefits and disadvantages to each type of training and especially to the difference between skills-based education and training versus the other methods that people might be normally thinking of as sort of the default?
Emmanuel: I think it depends on your current – Where you are currently in your career. If you’re sort of just starting out, I think it makes the most sense to do either a boot camp or a four-year degree. The reason I think boot camp actually might be better especially in cybersecurity is that – I mean, it's going to be faster you have guarantees and you can focus – You can get job skills as supposed to in a four-year degree where it's a little more roundabout, a little less direct, perhaps more expensive.
In this way, you are able to basically solve this neat experience to get experience paradox. A lot of people have difficulty entering cybersecurity despite the fact that we have a huge demand, which is very ironic. I think if you do a boot camp, that places you in a position where you have experience and now you are off to a great start. You can get more experience.
Now, I think once you are already in cybersecurity and you are pretty stable and you have specific requirements, then I think it makes sense to look at skills-based. Let's say hypothetically that I am a malware analyst and we are maybe having trouble with – I don’t know, Trojans, say, hypothetically. Then it makes sense in terms of time commitment to focus on Trojans as my skill. Understand how Trojans work, reverse them and dedicate that skill as supposed to taking malware analysis course more generally, which is not going to as in depth and is going to take longer, and you probably know it. If you're malware analysis, it's too general for you. I think like once you have a job or you have a project you’re working on, then you know what you need to drill into and then it makes sense to go skills-based, skills-based training. Of course, it never hurts to keep up the boot camps.
Chris: Right. Yeah, absolutely. Yeah, never stop learning. regarding the skills platform, I mean just to sort of for people who weren't quite sure what we’re talking about. You get access to X number of skill training courses that are sort of just – They’re kind of podcast. They’re just sort of floating out there and you pick and choose them as you need and work on them in your own pace. Without a professor or a boot camp instructor assigning weekly tasks, some find that it might be hard to stay on track and meeting your learning objectives. Do you have any tips to help lifelong learners stay focused on training and accomplish their goals when there aren't necessarily hard deadlines at the end of them?
Emmanuel: Yeah. That's a tough one. My thinking was it reminds me of this Jerry Seinfeld advice. He has this advice for when he was trying to work on his comedy skills. He wanted to make sure that he rides a joke each day. What he did was set up a calendar behind him on the wall and each day that he wrote a joke, he’d mark it a big red X, and basically as he kept doing it, he had like a chain of these big red Xs. At that point, he had this internal motivation not to break the chain, like don’t skip a day because then you will see that you’ve broke the chain.
Chris: That’s all you see in the calendar is that big red X.
Emmanuel: Yeah. I think this is a good advice for anyone learning skills. Maybe try to chop up your work into practical chunks that you can finish each day and then just don't break the chain.
Chris: There you go. What should you be shopping for when seeking out skills-based training? I mean, there's a lot of education programs out there, but what do you think distinguishes the best ones? What are the sort of products or services or the way they’re handled or whatever? What constitutes the best ones?
Emmanuel: I think that the best – It's really important that the education provider gives you a good experience. I think this is like a follow-up on the previous question in the sense that if you have a good experience in your course or in your skills-based training, then it's more likely that you will keep going, that you will keep coming back and you’ll keep learning, whereas if you had problems like customer service was bad or the platform crashed or whatever it is, then you kind of have this bad experience and you're less likely to keep going and you will break the chain. I think you want to make it easier for yourself to not break the chain and get quality education, somewhere where you’ll get good customer service, good experience and a good platform.
Chris: For people who might want to sign up for your class, and of course listeners of this podcast can be use promo code cyberwork to get a free month. What tips do you have for anyone who wants to get the most out of your classes specifically, and also once they’ve completed the coursework, what other areas of study would you recommend within the skills platform and sort of build at?
Emmanuel: Yeah. I would start with cyber security data science. There are two learning paths like I mentioned earlier, cybersecurity data science and then machine learning for red team hackers. The first one is the fundamentals. It's pretty hands-on, and I think that’s essential in cybersecurity that you work with real things, like you get real samples, you create a real lab, you create an actual classifier, because just reading about it, is not cannot going to help you. You’re not going to be able to do it in real-time when you need to.
I would suggest working with it. All the assignments are pretty handy. None of it is tedious. By the end of it, you’ll have experience with most of the important aspects of cybersecurity, like you will have worked with malware. You will have worked with phishing. You will have worked with intrusion detection and pentesting, social engineering, and now you’re pretty comfortably have a broad base.
Afterwards, if you are still motivated to focus on machine learning in particular, then you can go on to machine learning for red team hackers, which is I think really interesting. I mean, I personally think it's freaking so interesting to see how machine learning sees for offensive purposes. Then you can learn – You can go in-depth. This assumes that you finish cybersecurity data science and here you do things like deepfakes, fuzzing using machine learning and even attacks on like actual machine learning systems so that if you want to take your tools and your techniques to the next level, this is like the cutting edge of technology.
Once you’re there and you have these skills, then you focus on what you're specializing in, but you now have like a broad foundation from cybersecurity data science and then you know the cutting edge tools. So now it's just applying what you’ve learned.
Chris: Okay. One last question before we go. Where do you see deepfake technology going in the coming years? Do you think this is something that’s going to be with us for the immediate future or is this something that legislative agencies will eventually crackdown on or is this – Where do you see it going? Do you see it getting sort of more efficient, more used or do you see like an endpoint for this sort of thing?
Emmanuel: I think it's actually sort of like the way cybersecurity proceeds in a lot of ways. You kind of have like the hackers trying out like fringe new technologies and then they use it to like cause trouble. Then the security people catch up. They learn about these technologies and then they kind of use them for defense. I think there’s going to be a similar thing with deepfakes.
Right now, most government agencies, police and so on and so forth are not really employing deep learning technology too much, but we see like hackers utilizing these sort of tools to cause trouble, like for instance I mentioned the audio impersonation that led to defrauding by $250,000. Recently, motivated by these sorts of problems, the police are starting to employ deep learning technologies.
They are using this tool to basically figure out who a suspect is. Let's say that you are caught on camera burglarizing a car or whatever it is, and just because we have you on camera doesn't mean that we know who it is. It's hard to tell. They are now using deep neural networks to solve these sorts of crimes. So they look at the footage of the person committing the crime correlated with your social networks and other OSINT and maybe closed information, like government databases, and then we figure out, “Oh! Its Chris. He’s burglarizing cars again.”
Chris: It’s me again.
Emmanuel: Or something like that. I think in the near future, the government agencies and the police force are going to start utilizing the tools for defense and then it’s going to sort of equalize.
Chris: Do you see a point where the technology will catch up sufficiently in the Defense Department that it will make the concept of deepfakes obsolete?
Emmanuel: I think so. I think a lot of it is going to require other tools like crypto and awareness just that fake things exist, and then it’s going to become like just the special-effects tools, just like we have plenty in Hollywood. It's not that big of a concern. We know you can add in all sorts of – Like ways to beautify someone's face and it doesn’t, whereas we can Photoshop faces.
Chris: There you go. Okay, so one last question for today. If listeners want to know more about Emmanuel Tsukerman, where can they go online to find out about you?
Emmanuel: Sure. You can find me by adding me at LinkedIn. You can find my YouTube channel, and I think more interesting is, myself and my team, we write a monthly newsletter about cybersecurity data science. We basically find the most interesting and new things that are happening in cybersecurity and data science, the intersection of the two, and you can find it in a newsletter form. To sign up, you can go to ml4cs.com. Just the letters, the number 4 and then.com, and it's free and no spam.
Chris: Machine learning 4 – Yeah.
Emmanuel: Yeah.
Chris: Machine learning 4 computer security. Okay. All right. Emmanuel, thank you so much for your time today. I really appreciate it. This was very illuminating.
Emmanuel: It was a pleasure. I appreciate it.
Chris: Thank you all today for listening and watching. If you enjoyed today's video, you may find many more on our YouTube page. Just go to youtube.com and type in Cyber Work with Infosec to check out a collection of tutorials, interviews and past webinars. If you'd rather have us in your ears during your workday, all of our videos are also available as audio podcasts. Just search Cyber Work with Infosec in your podcast catcher of choice.
If you wouldn't mind, please consider rating and reviewing and liking our videos and so forth. It really does help out give us bump what people know. For a free month of the Infosec skills platform that we discussed in today's show, just go to infosecinstitute.com/skills and sign up for an account. There’ll be a coupon code there and podcast listeners can type in the word cyberwork, all one word, all small letters, no spaces, and you'll get a free month.
Thank you once again to Emmanuel Tsukerman, and thank you all for watching and listening. We will speak to you next week.
Subscribe to podcast
How does your salary stack up?
Ever wonder how much a career in cybersecurity pays? We crunched the numbers for the most popular roles and certifications. Download the 2024 Cybersecurity Salary Guide to learn more.
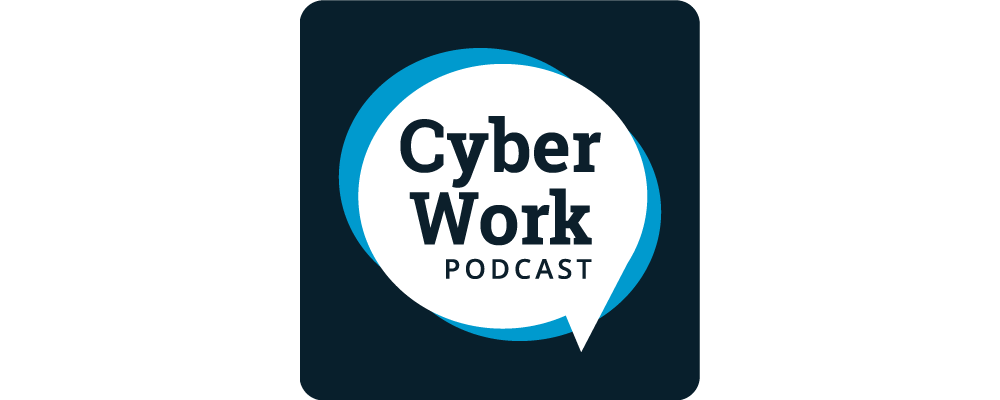
Weekly career advice
Learn how to break into cybersecurity, build new skills and move up the career ladder. Each week on the Cyber Work Podcast, host Chris Sienko sits down with thought leaders from Booz Allen Hamilton, CompTIA, Google, IBM, Veracode and others to discuss the latest cybersecurity workforce trends.
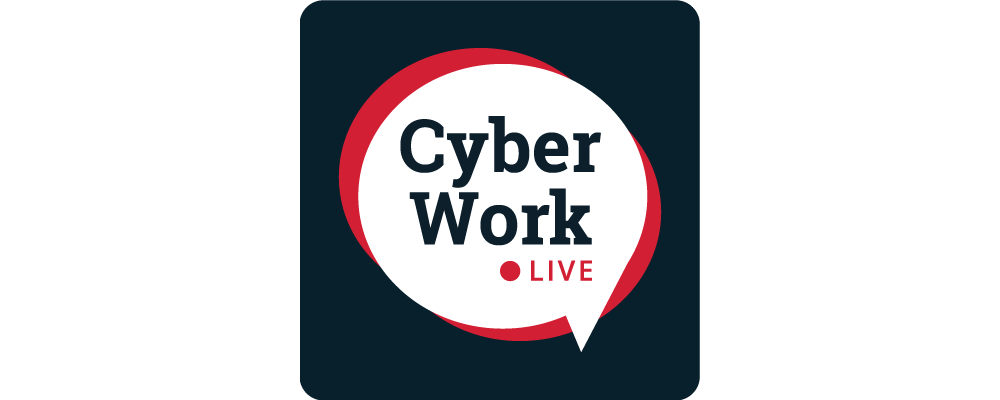
Q&As with industry pros
Have a question about your cybersecurity career? Join our special Cyber Work Live episodes for a Q&A with industry leaders. Get your career questions answered, connect with other industry professionals and take your career to the next level.
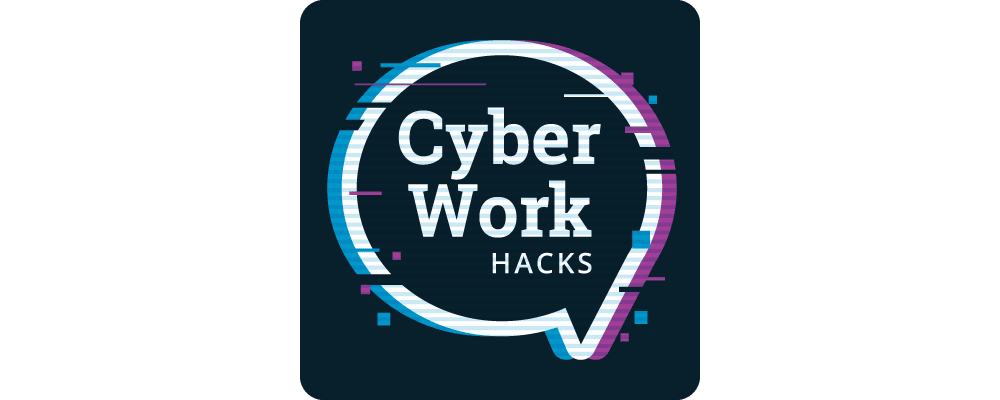
Level up your skills
Hack your way to success with career tips from cybersecurity experts. Get concise, actionable advice in each episode — from acing your first certification exam to building a world-class enterprise cybersecurity culture.